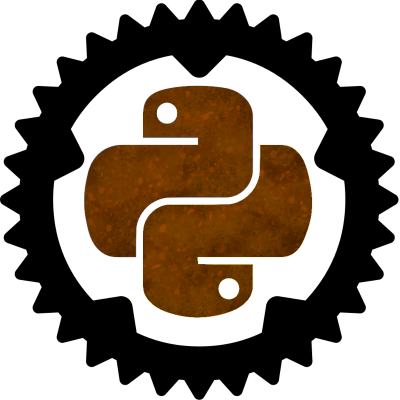
What to expect from this blog post Bootstrapping the extension module Brief introduction to PSBTs Building a simple PSBT with Rust Type hints for our native class Conclusion What to expect from this blog post This blog post is meant to be an introduction to PyO3 by walking the reader through the build process of a non-trivial extension module in Rust using PyO3. Some familiarity with Python and Rust is recommended to get the most out of this post, basic understanding of Bitcoin concepts may be required to fully grasp the code samples.
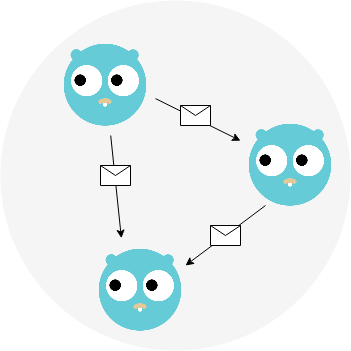
Go 1.18 is scheduled to release in February 2022, this release is a big one because it introduces the highly anticipated (and controversial) Type Parameter feature aka generics; it’s probably going to take some time until we actually migrate to 1.18 at work, so I’ve been looking for excuses to play with it.
A few weeks ago I worked on a feature that could have been implemented succinctly using the actor model, after thinking it through, I realized generics would make it possible to have actors without relying on interface{} too much so I decided to play with how the API could look like.
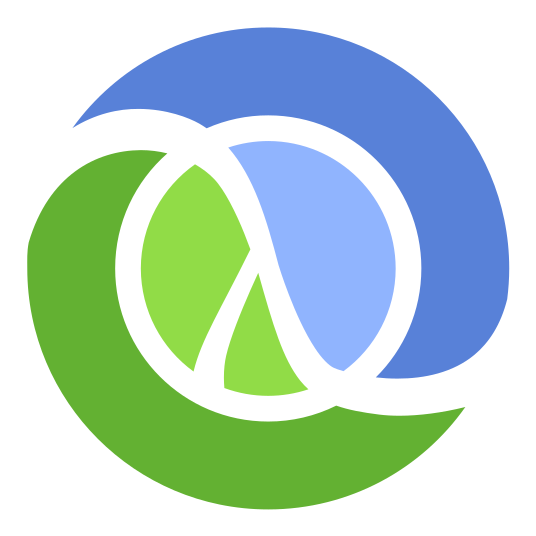
I remember the first time I read about the book “Seven languages in seven weeks”, back then I was still a student working on my graduation project but even then I was able to see the value in exploring seven languages in such a short amount of time. For one reason or another I ended up not reading it at the time, I just added it to an ever growing list of “things I’ll eventually get back to”.
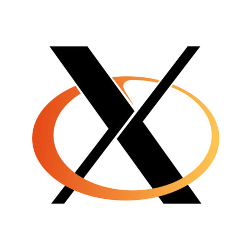
Recently I partcipated in a project that involved some server-side GPU rendering, due to the nature of the technologies we used we needed to run an X server on our boxes. The initial setup was relatively painless until a weird bug started appearing in some server and the logs didn’t provide enough information to debug effectively, this is how I ended up coding a remote viewer that streamed a remote server “screen” using WebRTC.
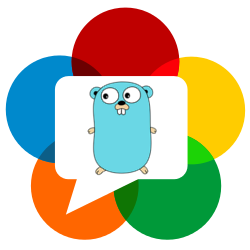
I’ve developed quite a few WebRTC applications over the last three years and noticed that as ML-based features arrive on almost every popular application one way or another, there’s been an increase on feature requests that involve speech to text, sentiment analysis, natural language understanding and computer vision.
Almost every time one of these features is implemented it requires a considerable amount of engineering resources, probably because the available tools have a steep learning curve or aren’t a good match for the specific use case.